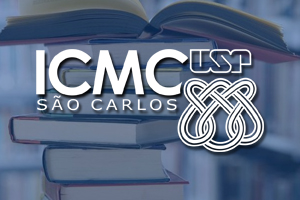
Acesso virtual: https://hangouts.google.com/hangouts/_/icmc.usp.br/grupomvl)
Resumo:
One of the most used methodologies in the field of education assessment is Item Response Theory (IRT). High dimensional latent space is still a challenge for usual estimation methods in IRT models, like MCMC or marginal maximum likelihood. In this work, we propose the use of a novel Variational Autoencoder (VAE) architecture, a kind of unsupervised deep neural network, for a multidimensional IRT model. Our approach combines the advantages of the IRT model with the flexibility of the VAE methodology, allowing us to estimate high latent trait dimensions and partially interpret the neural network.
The simulation studies show that, given enough data, the proposed method is competitive with the state-of-the-art ones with respect to predictive power and is much faster in runtime performance.